Next: Noise Model Comparison
Up: tr03mw2
Previous: Activation Height Modelling
The overall objective is to obtain the joint posterior
distribution of all unobserved parameters in the model, given the
observed data. Analytical approaches are not possible with
complicated models such as those being considered in this work.
Whilst it is possible to perform approximations to the
distribution, it is difficult to assess the effect of these
approximations on the inference performed. Therefore, the approach
taken instead is to use Markov Chain Monte Carlo (MCMC) sampling
from the full joint posterior distribution (see (22) and
(20) for texts on MCMC).
We are able to use Gibbs sampling for all noise parameters. For
the signal parameters (the activation height and HRF parameters)
we use single-component Metropolis-Hastings jumps (i.e. we propose
separate jumps for each of the parameters in turn). We use Normal
proposal distributions with the mean fixed on the current value,
and with a scale parameter
for each parameter that is
updated every 30 jumps. At the
update
is
updated using:
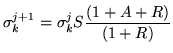 |
|
|
(22) |
where
and
are the number of accepted and rejected jumps
since the last
update respectively,
is the desired
rejection rate, which we fix at
(22).
When appropriate, parameters are initialised using ordinary least
squares. We use a burn-in of 2000 jumps, followed by 2000 further
jumps of which every 4th is sampled. Observation of the chains
with different (but still sensible) initial conditions confirmed
that a burn-in of 2000 samples was sufficient. The HRF parameters
are initialised to the middle of their ranges. Details of the
sampling used for each of the different parameters are described
in the appendix.
Next: Noise Model Comparison
Up: tr03mw2
Previous: Activation Height Modelling