Next: FMRI data
Up: Artificial data
Previous: Methods
Figures 3--7 show the
results of inferring on the three different continuous weights
mixture models on the five different artificial datasets. The
spatial maps in the figures are unthresholded marginal posterior
means of
, i.e.
, for all three
classes of deactivation, non-activation and activation.
We can compute the effective global
class proportions based upon the classifications,
i.e.:
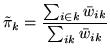 |
(25) |
where the weights
are the mean marginal posterior weights.
These effective global
class proportions can be combined with the mean marginal posterior
class distribution parameters to give a histogram fit. These are
shown in figures 3--7(a).
The box plot in figure 8 shows
the marginal posterior distributions of the
MRF smoothness parameter
for the different
artificial datasets.
The value of
clearly varies a lot from dataset to dataset
emphasising the need for adaptive determination of the spatial smoothness.
It is interesting to compare model 2's
performance with that of model 3.
Model 3 is the same as model 2, except that in model 3
the spatial regularisation parameter is adaptive and in model 2 it is fixed.
Model 2 works well on some datasets, for example figures 6 and 7. This is because the arbitrarily chosen
value of
is close to the adaptively determined
values of
for those datasets,
which can be seen in figure 8 (note that
).
For the other datasets it works less well. For example, it overblurs the
edges in figure 3 and overblurs the activation
and deactivation to the point of removing much of it in figures 4 and 5.
It is worth emphasising that model 3 also works well on
the no activation dataset (figure 7). This is
a dataset where there is in fact only one class present (the non-activation
class), and yet we fit the mixture model assuming three classes.
Despite this, model 3 forces
all probabilities to well less than
for the activation and
deactivation classes.
Figure 3:
Results for the large activation artificial dataset.
Top left is the actual data,
.
(a) shows the histograms of
along with the fit
for the different mixture models (the red lines show the individual
class distributions and the dashed yellow line shows the overall
fit).
(b) Spatial maps of unthresholded
for [left] deactivation
[middle] non-activation [right] activation.
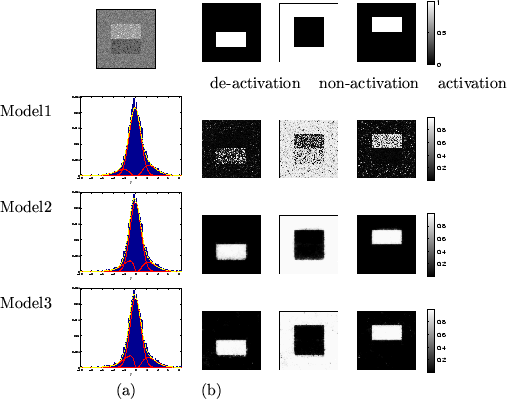 |
Figure 4:
Results for the small activation artificial dataset.
Top left is the actual data,
.
(a) shows the histograms of
along with the fit
for the different mixture models (the red lines show the individual
class distributions and the dashed yellow line shows the overall
fit).
(b) Spatial maps of unthresholded
for [left] deactivation
[middle] non-activation [right] activation.
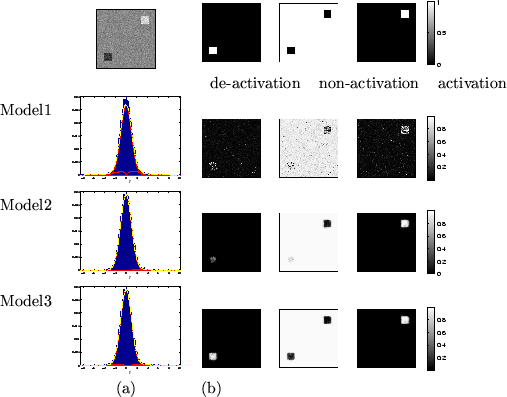 |
Figure 5:
Results for the random checker activation artificial dataset.
Top left is the actual data,
.
(a) shows the histograms of
along with the fit
for the different mixture models (the red lines show the individual
class distributions and the dashed yellow line shows the overall
fit).
(b) Spatial maps of unthresholded
for [left] deactivation
[middle] non-activation [right] activation.
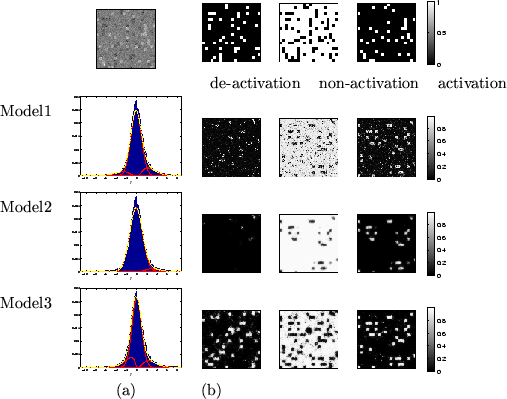 |
Figure 6:
Results for the Gaussian activation artificial dataset.
Top left is the actual data,
.
(a) shows the histograms of
along with the fit
for the different mixture models (the red lines show the individual
class distributions and the dashed yellow line shows the overall
fit).
(b) Spatial maps of unthresholded
for [left] deactivation
[middle] non-activation [right] activation.
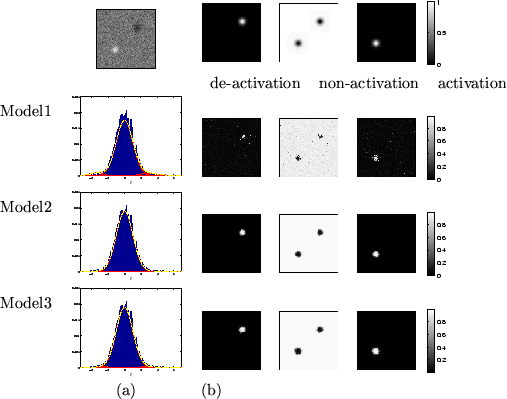 |
Figure 7:
Results for the no activation artificial dataset.
Top left is the actual data,
.
(a) shows the histograms of
along with the fit
for the different mixture models (the red lines show the individual
class distributions and the dashed yellow line shows the overall
fit).
(b) Spatial maps of unthresholded
for [left] deactivation
[middle] non-activation [right] activation.
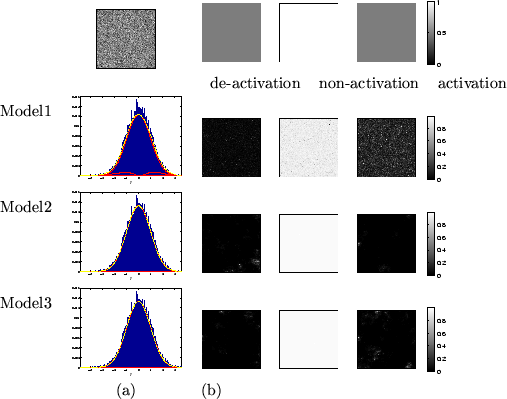 |
Figure:
Box plot showing the marginal posterior distributions of the
MRF smoothness parameter
for the different datasets.
The value of
clearly varies a lot from dataset to dataset
emphasising the need for adaptive determination of the spatial smoothness.
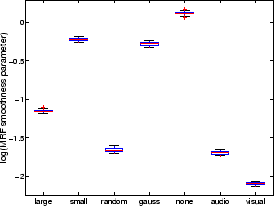 |
Next: FMRI data
Up: Artificial data
Previous: Methods