Next: Discussions
Up: Segmentation of Brain MR
Previous: HMRF-EM Framework for Brain
Various experiments have been carried out both on real and
simulated data, in both 2D and 3D. For the MEM algorithm, the
parameters used in the experiments take their actual values for
the simulated images and are manually estimated for real data,
since the algorithm does not deal with parameter estimation
itself. For the HMRF-EM algorithm, parameters are estimated
automatically.
The first experiment shown here tests the noise sensitivity of the
two algorithms. Two images consisting of two constant regions with
the same simulated bias field but with different white noise were
generated (Figure 6(a),(b)). Two Gaussian classes,
corresponding to the two regions, are used. For Figure
6(a), both algorithms give perfect estimation results, as
shown in Figures 6(c) and (d). However, for Figure
6(b), the HMRF-EM algorithm gives much better results than
the MEM algorithm.
Figure 6:
Comparison of the MEM and the HMRF-EM algorithm on
simulated 2D images. (a) the original image with 3% noise. (b)
the original image with 10% noise. (c) bias field estimation for
(a) by both the algorithms. (d) segmentation for (a) by both the
algorithms. (e) bias field estimation for (b) by the MEM
algorithm. (f) segmentation for (b) by the MEM algorithm. (g) bias
field estimation for (b) by HMRF-EM algorithm. (h) segmentation
for (b) by the HMRF-EM algorithm.
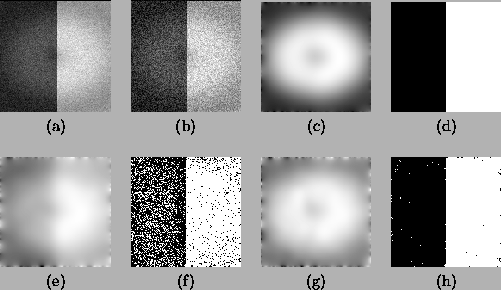 |
The second experiment tests the performance of the two algorithms
on real 2D MR images but with a simulated bias field. Two Gaussian
distributions are used for the two tissue classes (white matter
and grey matter) and a uniform distribution (density = 0.3) is
used for the rest. Figure 7(a) is the original
T2-weighted image and Figure 7(b) is the image with a
simulated circular bias field. Figure 7(c) is the
histogram of Figure 7(b), from which a substantial
intensity overlap between WM and GM can be seen. Figure
7(d) shows the best result that can be obtained from (a)
using global thresholding. The second row shows the result of
applying the MEM algorithm. The last row shows the results from
the HMRF-EM algorithm. Comparing the segmentations, we see that
without losing any significant structure, the results from the
HMRF-EM algorithm are much cleaner than from the MEM method, which
still looks noisy. The restored image from HMRF-EM algorithm also
shows good intensity uniformity, while the histogram output in the
MEM method still shows WM/GM overlap.
Figure 7:
Comparison of the MEM and the HMRF-EM algorithm on real
2D MR images with simulated bias field. (a) the original image;
(b) the image with simulated bias field; (c) histogram of (b); (d)
best thresholding on (b); (e)-(h) the results from the MEM
algorithm; (i)-(l) the results from HMRF-EM algorithm. For the
last two rows, from left to right: the estimated bias field (the
checkerboard is used to represent the background which is assumed
to have no bias field), the segmentation, the restored image and
the histogram of the restored image.
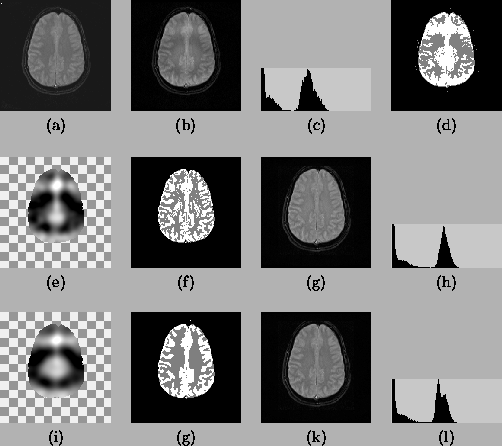 |
The last example we show is for a real 3D data set from the
Montreal Neurological Institute, McGill University, courtesy of D.
Arnold. The original volume has 50
slices with
voxel size
mm. Figure 8
shows the results of five different slices from the HMRF-EM
algorithm.
Figure 8:
Five slices of a 3D MR volume image with real bias field.
In each row, from left to right: the original slice, the estimated
bias field, the restored slice, and the segmentation.
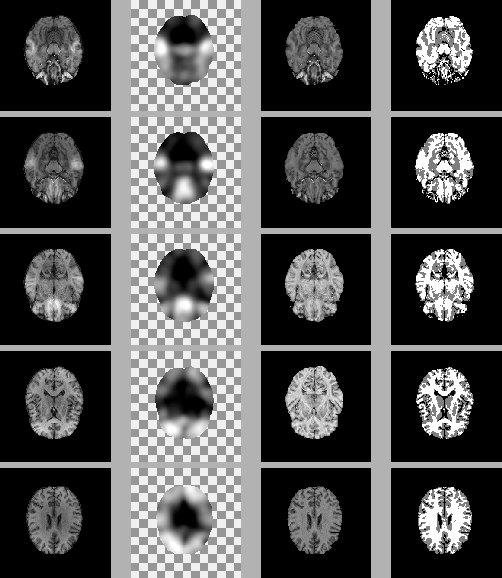 |
Next: Discussions
Up: Segmentation of Brain MR
Previous: HMRF-EM Framework for Brain
Yongyue Zhang
2000-05-11