Next: Non-spatial with Class Proportions
Up: Discrete Labels Mixture Model
Previous: Discrete Labels Mixture Model
We assume spatial independence between the classification
labels,
, at each voxel:
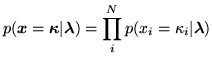 |
(2) |
and that the prior on the discrete classification labels,
,
is a non-informative distribution with each class having equal
probability. Using this in equation 1,
the posterior becomes:
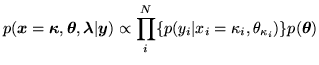 |
(3) |