Next: Basis Functions
Up: Model
Previous: Bayesian framework
Autoregressive Parameters Spatial Prior
In Penny et al. (2003) non-spatial non-informative priors were used on
the autoregressive parameters. Previous work has shown that
neighbouring voxels have similar temporal
autocorrelation (Woolrich et al., 2001; Worsley et al., 2002). Therefore, we want
to model the assumption that a priori we expect neighbouring
voxels to have similar temporal autocorrelation. To do this we use
a Gaussian conditionally specified auto-regressive (CAR) or
continuous Markov Random Field (MRF) prior (Cressie, 1993) on
each of the
autoregressive parameter maps, i.e.
with:
 |
(7) |
where
denotes a multivariate Normal distribution,
is
an
matrix whose (i,j)th element is
, and
is the MRF control parameter which controls the
amount of spatial regularisation. We set
,
if
and
are spatial neighbours and
otherwise (where
is the geometric mean of the
number of neighbours for voxels
and
). We also require a
hyperprior on
, for which we use a standard
noninformative conjugate gamma prior:
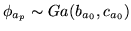 |
(8) |
Before we specify the prior for the regression parameters
,
we consider how we can use basis functions within this framework.
Next: Basis Functions
Up: Model
Previous: Bayesian framework