Next: Results
Up: Effect of Different Regressors
Previous: Effect of Different Regressors
It turns out that the regressor used in the design matrix
considerably affects the relative efficiency between
colouring and prewhitening.
To illustrate this we use a typical autocorrelation
estimated for a grey-matter voxel (shown in figure 5) to give
a typical
matrix, and use for
one of four different types of regressors of particular interest:
- (a)
- a boxcar design with period 60 secs
- (b)
- a single-event (SE) design with fixed inter-stimulus interval (ISI)
of 15 secs and stimulus duration of 0.1 secs
(Bandettini and Cox, 2000)
- (c)
- a single-event design with stimulus duration of 0.1 secs with jittering
such that the ISIs are drawn from a uniform distribution U(13.5 secs, 16.5 secs)(Josephs et al., 1997)
- (d)
- a single-event design with randomized ISI
taken from a normal distribution with mean
and standard
deviation
with no ISI less than
(Burock et al. (1998),
Dale and Buckner (1997) and Dale (1999)) and stimulus duration of 0.1 secs.
All four designs are convolved with the same gamma HRF:
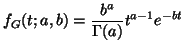 |
(19) |
where the Gamma parameters
are set according to mean
and variance
. The Gamma HRF for these parameters
is shown in figure 6.
More complicated HRF models could be used. However, any reasonable HRF model
would be expected to have a similar spectral density and therefore behave in a
similar way in this context.
For all regressors TR is taken as 3 secs and all regressors have their means removed.
Figure 5:
(a) Autocorrelation for a typical grey-matter voxel in a rest/null
data set, and (b) the power spectral density for the same autocorrelation.
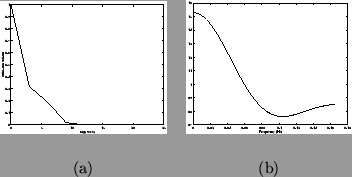 |
Figure 6:
(a) Gamma HRF,
has parameters set according to
mean
and variance
, and (b) the spectral density for the HRF.
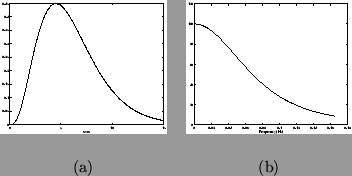 |
The variance of the parameter estimates,
, is
inversely proportional to the efficiency and can give us a measure
of the relative efficiency of the different temporal filtering
strategies. Although the estimation of
does depend upon the
temporal filtering strategy used (equation 4 depends
on
), this effect is negligible.
Subsequently, we define a measure of
efficiency,
, relative to
the maximally efficient prewhitening estimator for the regressor as:
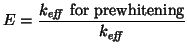 |
(20) |
This was computed for each regressor using
each of the three different temporal filtering strategies using
equations 6, 7, 8 accordingly
(there is only one regressor in each case so we use
).
The low pass filter used for the colouring
was matched to the HRF (figure 6).
The values of
for the randomised ISI and jittered ISI designs
were averaged over
randomly generated designs.
Next: Results
Up: Effect of Different Regressors
Previous: Effect of Different Regressors
Mark Woolrich
2001-07-16