Next: FMRI data
Up: tr04mw2
Previous: Results
Spatial Mixture Modelling
Using Variational Bayesian inference we have produced a spatial
map of pseudo-z-statistics. Since the pseudo-z-statistics for the
constrained HRF model give smaller empirical null distribution
probabilities than the nominal FPR, we could use the
pseudo-z-statistics and infer on them using frequentist
probabilities without violating our nominal FPR. However, we would
not be taking full advantage of the extra sensitivity of the
Bayesian inference when we have informative priors constraining
the HRF shape.
Therefore, we look to infer on the spatial map of
pseudo-z-statistics using the technique of spatial mixture
modelling. In mixture modelling we model the spatial map as being
made up of voxels which are classified as either activating or
non-activating (Everitt and Bullmore, 1999). The activation and deactivation
classes have different distributions which describe
probabilistically the pseudo-z-statistic values we expect in that
class. Since we are estimating the non-activating distribution
from the data we can adjust to the shifted non-activation
distribution demonstrated in figure 5.
In spatial mixture modelling we augment this histogram information
with spatial regularisation of the
classification (Hartvig and Jensen, 2000; Woolrich et al., 2004a). Note that this has
nothing to do with the way in which we spatially regularise the
temporal autocorrelation coefficients in section 2.2.
Instead, we a spatially regularising the classification of
activating or non-activating voxels. This has been implemented
in Woolrich et al. (2004a), within a completely adaptive fully Bayesian
framework. Importantly, this approach adaptively determines the
amount the classification is spatially regularisation.
Figure 6 shows the null distribution of
pseudo-z-statistics resulting from the constrained HRF analysis on
the artificial null data in section 4. This
distribution is not Normal, in particular it is asymmetric. We
therefore model the non-activation distribution in the mixture
modelling as a flipped and shifted Gamma distribution.
Figure 6 shows the fit of a flipped and
shifted Gamma distribution to the null distribution histogram.
Recall that with an f contrast we only look at the positive tail
of the distribution to find both activations and deactivations.
Therefore, we model the activations and deactivations as a single
class in the spatial mixture modelling using a straightforward
Gamma distribution.
Figure 6:
Null
distribution of pseudo-z-statistics resulting from the constrained
HRF analysis on the artificial null data in
section 4. This is the same histogram as that shown
in red in figure 5(a). Also shown is the
fit to this histogram of a flipped and shifted Gamma
distribution.
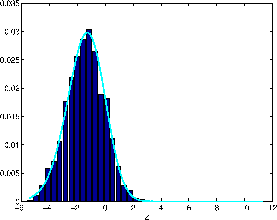 |
Next: FMRI data
Up: tr04mw2
Previous: Results